the Creative Commons Attribution 4.0 License.
the Creative Commons Attribution 4.0 License.
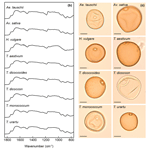
Chemotaxonomy of domesticated grasses: a pathway to understanding the origins of agriculture
Phillip E. Jardine
William D. Gosling
Barry H. Lomax
Adele C. M. Julier
Wesley T. Fraser
The grass family (Poaceae) is one of the most economically important plant groups in the world today. In particular many major food crops, including rice, wheat, maize, rye, barley, oats and millet, are grasses that were domesticated from wild progenitors during the Holocene. Archaeological evidence has provided key information on domestication pathways of different grass lineages through time and space. However, the most abundant empirical archive of floral change – the pollen record – has been underused for reconstructing grass domestication patterns because of the challenges of classifying grass pollen grains based on their morphology alone. Here, we test the potential of a novel approach for pollen classification based on the chemical signature of the pollen grains measured using Fourier transform infrared (FTIR) microspectroscopy. We use a dataset of eight domesticated and wild grass species, classified using k-nearest neighbour classification coupled with leave-one-out cross validation. We demonstrate a 95 % classification success rate on training data and an 82 % classification success rate on validation data. This result shows that FTIR spectroscopy can provide enhanced taxonomic resolution enabling species level assignment from pollen. This will enable the full testing of the timing and drivers of domestication and agriculture through the Holocene.
- Article
(3504 KB) - Full-text XML
-
Supplement
(780 KB) - BibTeX
- EndNote
The transition from a mobile, hunter–gatherer lifestyle to a sedentary lifestyle centred on agriculture was one of the major shifts in the history of human civilisation. While many different species of plants were successfully domesticated over the course of the Holocene, grasses (Poaceae) have been a particular focus of exploitation, both for human consumption and as animal feed. Principal grass crops include wheat (Triticum), rice (Oryza), maize (Zea), barley (Hordeum), rye (Secale), oats (Avena), Sorghum, sugarcane (Saccharum), and the millets (principally Eleusine, Panicum, Pennisetum and Setaria, but also including other genera from within the subfamilies Chloridoideae and Panicoideae) (Fig. 1a) (Kellogg, 1998; Leff et al., 2004; Meyer et al., 2012), which together account for ∼63 % of total cultivated land area (Leff et al., 2004). Understanding the initial exploitation and domestication of grasses and the spread of these lineages and agricultural practices is therefore a key research endeavour, including questions of when, where and why particular lineages were domesticated and what impact this had on human societies and the landscapes in which they lived. A fuller understanding of domestication may also play an important role in securing future food security (Charmet, 2011).
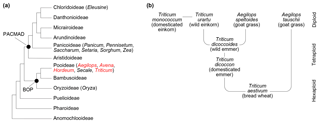
Figure 1(a) Poaceae subfamily cladogram, redrawn from Soreng et al. (2015). BOP clade: subfamilies Bambusoideae, Oryzoideae and Pooideae. PACMAD clade: subfamilies Panicoideae, Aristidoideae, Chloridoideae, Micrairoideae, Arundinoideae and Danthonioideae. The genus names in parentheses are crop taxa mentioned in the text, and those in red are included in this study. (b) Hybridisation and domestication pathway for wheat, showing relationships among taxa and their ploidy levels. All taxa except Aegilops speltoides are included in this study (see also Table 1). Redrawn from International Wheat Genome Sequencing Consortium (2014), with additional information from Petersen et al. (2006).
Evidence for the domestication of grasses and the spread of agriculture has come from a variety of sources. A combination of modern and ancient DNA analysis (Dvorak et al., 2006; International Wheat Genome Sequencing Consortium, 2014; Marcussen et al., 2014; Mascher et al., 2016; Meyer and Purugganan, 2013; Petersen et al., 2006), controlled growth experiments (Cunniff et al., 2014; Preece et al., 2015, 2017, 2018), and archaeobotanical remains of grasses and grain processing (Crowther et al., 2016; Fuller, 2007; Piperno et al., 2004; Savard et al., 2006; Vignola et al., 2017; Weiss et al., 2004; Willcox et al., 2007) have together provided a detailed picture of when and where different taxa were domesticated, including the hybridisation pathways that produced the modern domesticated types (Fig. 1b), and why certain species may have been selected for cultivation over others. A key outstanding challenge is the need for better spatially and temporally resolved data on the first appearances of domesticated species and their subsequent diffusion to other regions, which will in turn enable a fuller understanding of the role that climatic and environmental changes played in shaping the origins and spread of agriculture (Larson et al., 2014).
Of the different sources of empirical evidence for historical vegetation change, pollen provides the most abundant and widespread records, but is also one of the most challenging to utilise for tracking grass domestication and agricultural practices through time. This is because pollen morphology is highly similar across the ∼12 000 species (Soreng et al., 2015) within Poaceae (Strömberg, 2011). Poaceae pollen grains are all morphologically simple, being more or less spherical with a single, annulate pore and a scabrate to areolate surface sculpture (Köhler and Lange, 1979; Mander et al., 2013). Attempts to distinguish among and between wild grasses and their domesticated relatives have relied on a combination of grain size and shape, pore diameter and position, annulus width and thickness, and exine structure and microsculpture (Andersen, 1979; Beug, 1961, 2004; Bottema, 1992; Dickson, 1988; Firbas, 1937; Joly et al., 2007; Köhler and Lange, 1979; Rowley, 1960; Tweddle et al., 2005), although of these various characters grain size has been most commonly relied upon in routine palynological studies (Bottema, 1992). Pollen grain size varies between 30 and 100 µm among Poaceae species and broadly correlates with genome size (Bennett, 1972). Since domesticated grasses are typically polyploid they have larger pollen grains than their wild type relatives, and this has led to a size-based circumscription of a “Cerealia” type (Andersen, 1979; Beug, 1961, 2004; Bottema, 1992; Firbas, 1937; Joly et al., 2007; Tweddle et al., 2005). In practice, however, there is often an overlap in size between wild and domesticated pollen types, especially outside of northwest Europe where wild grasses frequently exceed the 40 µm Cerealia size cut-off (Bottema, 1992; Joly et al., 2007; Tweddle et al., 2005). Pollen size is also known to be influenced by processing procedures, and storage and mounting media, with both acetolysis and storage in glycerol leading to a size increase (Christensen, 1946; Cushing, 1961; Faegri and Deuse, 1960; Reitsma, 1969; Sluyter, 1997; see also Jardine and Lomax, 2017, for review).
Variations in pollen grain surface sculpture have been used as a basis for dividing grains up into broad types, such as the Hordeum type, Triticum type, Avena type and Setaria type of Köhler and Lange (1979). Additional information on grain morphology has then been used to separate out individual taxa, such as Secale cereale L. (rye) being distinguished from other members of the Hordeum type by grain shape and pore position (Dickson, 1988; Köhler and Lange, 1979). These sculpturing types are, however, not phylogenetically or taxonomically meaningful and occur across the grass phylogeny (Mander and Punyasena, 2016). Triticum monococcum (domesticated einkorn) has been assigned to the Hordeum rather than the Triticum type based on scanning electron microscopy (SEM) analysis of its exine microsculpture (for example Köhler and Lange, 1979), and the Hordeum, Triticum, Avena and Setaria types all occur in both the PACMAD and BOP clades (Fig. 1a) (Mander and Punyasena, 2016). Surface and structural micro-features are also challenging to unambiguously identify using light microscopy, even if high magnification and/or phase contrast are employed (Andersen and Bertelsen, 1972; Mander et al., 2013; Rowley, 1960; Tweddle et al., 2005). While sophisticated computational image-based classification approaches have been developed on Poaceae pollen (Mander et al., 2013) they have not yet been applied to crop domestication problems, and since they rely on SEM imaging would be costly and time consuming to perform on the large numbers of specimens and samples required to make meaningful insight into the topic (Julier et al., 2016). Finally, all size, shape and sculpturing characters may be influenced by taphonomy (Bottema, 1992; Jardine and Lomax, 2017), and it is not clear how useful they are, even in combination, for examining the earliest phases of wild grass hybridisation and domestication.
Recent research has demonstrated the potential of using the chemical signature of pollen grains as an alternative means of classification. Non-destructive vibrational spectroscopic methods such as Fourier transform infrared (FTIR) and Raman spectroscopy have been applied to pollen and spore classification problems in both plants and fungi (Bağcıoğlu et al., 2015; Dell'Anna et al., 2009; Ivleva et al., 2005; Julier et al., 2016; Pappas et al., 2003; Schulte et al., 2008; Zimmermann, 2010, 2018; Zimmermann and Kohler, 2014; Zimmermann et al., 2015a, b, 2016) and have demonstrated a high degree of accuracy in discriminating between closely related taxa. Zimmermann (2018) applied FTIR to chemically profile and separate two morphological similar species of pine (Pinus mugo Turra and Pinus sylvestris L.). A further FTIR study based on grasses of tropical West Africa (Julier et al., 2016) revealed a subfamily level classification success rate of 80 %, suggesting that this approach might be useful for distinguishing among different species of domesticated and wild grasses. Here we test this possibility on a dataset of grass crops and their wild progenitors, with a view to developing pollen chemotaxonomy as a viable archaeobotanical tool.
Anthers from eight species of domesticated and wild grasses (Table 1) were collected from the John Innes Centre, Norwich, UK (52.62∘ N, 1.22∘ E), in May and June 2014. All species are from the subfamily Pooideae (Fig. 1a), with Triticum (wheat), Aegilops (goat grass) and Hordeum (barley) from the tribe Triticeae and Avena (oat) from the tribe Poeae. The Triticum and Aegilops plants were grown outdoors as part of the bread wheat domestication demonstration at the John Innes Centre, and the Avena and Hordeum plants were grown and sampled in greenhouses. We sampled three plants from each species, except for T. monococcum (domesticated einkorn) where only two plants were in anthesis at the time of sampling. Four to six anthers were collected from each plant and combined together to give a sufficient quantity of pollen for chemical analysis. This means that each sample represents a whole plant, rather than an individual anther.
We used Fourier transform infrared (FTIR) microspectroscopy to generate chemical data, because it is an efficient method that has demonstrated success in pollen characterisation and classification studies (Bağcıoğlu et al., 2015, 2017; Bell et al., 2018; Bernard et al., 2015; Dell'Anna et al., 2009; Depciuch et al., 2018; Domínguez et al., 1998; Fraser et al., 2012, 2014; Gottardini et al., 2007; Jardine et al., 2015, 2017; Julier et al., 2016; Lomax et al., 2008; Pappas et al., 2003; Steemans et al., 2010; Watson et al., 2007; Zimmermann, 2010, 2018; Zimmermann and Kohler, 2014; Zimmermann et al., 2015a, b, 2016). The pollen samples were picked onto ZnSe windows for FTIR analysis, which was carried out using a Thermo Scientific (Waltham, MA, USA) Nicolet Nexus FTIR bench unit, attached to a Continuum IR microscope fitted with an MCT-A liquid nitrogen-cooled detector, run in transmission mode using a Reflachromat 15× objective. To remove atmospheric H2O and CO2 interference within spectra, the entire system (bench unit, microscope and sample stage) was purged with air that has been dried and scrubbed of CO2 using a Peak Scientific (Billerica, MA, USA) ML85 purge unit. We collected eight replicate spectra from each sample using an aperture size of 100 µm × 100 µm at 256 scans per replicate and a resolution of 4 cm−1. A background scan of a blank region of the ZnSe window was taken before each sample scan.
Data analyses were carried out in R v.3.4.2 (R Core Team, 2017) using the packages baseline v.1.2-1 (Liland and Mevik, 2015), caret v.6.0-77 (Kuhn, 2017), class v.7.3-14 (Venables and Ripley, 2002), corrplot v.0.84 (Wei and Simko, 2017), e1071 v.1.6-8 (Meyer et al., 2017) and prospectr v.0.1.3 (Stevens and Ramirez-Lopez, 2013). Although some pollen chemistry studies (e.g. Bağcıoğlu et al., 2015, 2017; Zimmermann and Kohler, 2014) have cropped FTIR spectra to the 1900 to 700 cm−1 region (i.e. the “fingerprint” region), preliminary analysis showed that with our dataset this decreased classification success by 1 % to 2 %. While this suggests that the majority of relevant information for classification is in the fingerprint region, we proceeded with the full 4000 to 690 cm−1 range to maximise classification success and analyse inter-taxon differences across the entire spectra. We corrected baseline drift by subtracting a second-order polynomial baseline from each spectrum. Variations in sample thickness will control the absolute height of spectra, so each spectrum was z score standardised by subtracting the mean value and dividing by the variance. We used principal component analysis (PCA) and cluster analysis for data visualisation and exploration (Varmuza and Filzmoser, 2009). The PCA was run on the sample spectra, and the cluster analysis was run on the mean spectrum for each taxon, with the aim of exploring the main inter-taxon chemical relationships. The cluster analysis was run using the Euclidean distance and with the unweighted pair group method with arithmetic mean (UPGMA) linkage algorithm.
To test the classification potential of the chemical data, the dataset was split randomly into training (two-thirds of the samples) and validation (one-third of the samples) subsets. For the taxa with three plants sampled this involved selecting one plant at random and assigning the spectra from it to the validation set. For T. monococcum, where only two plants were sampled, 5 of the 16 spectra were randomly selected and assigned to the validation set.
We used k nearest neighbour (k-nn) classification, which assigns unknown spectra to groups (species) based on the most chemically similar spectra (the “nearest neighbours”) from the training set (Julier et al., 2016; Varmuza and Filzmoser, 2009). The parameter k is the number of nearest neighbours used for classification and is user selected. Where k>1 the classification is determined by majority vote, and in the case of a tie the group assignment is chosen at random (Venables and Ripley, 2002). We used the Euclidean distance measure to determine between-sample chemical similarity.
In addition to running the k-nn classification on the unprocessed IR spectra, we tested the impact of Savitzky–Golay smoothing and taking first and second derivatives of the smoothed spectra. Savitzky–Golay smoothing fits polynomial curves to successive windows across a data series, with a larger window size increasing the amount of smoothing (Julier et al., 2016; Varmuza and Filzmoser, 2009). Taking derivatives of the spectra can bring out smaller spectral details to aid in classification but also increases the level of noise in the analysis (Julier et al., 2016; Varmuza and Filzmoser, 2009) and so was only carried out on the Savitzky–Golay smoothed spectra.
We used leave-one-out cross validation (LOOCV) on the training dataset to find the best combination of parameters for classification. LOOCV treats each spectrum as an unknown sample and classifies it based on the group membership of the rest of the dataset. The classification success rate can then be calculated as the percentage of correct classifications from the cross validation procedure (Varmuza and Filzmoser, 2009). We varied k (the number of nearest neighbours in the k-nn classification) and w (the window size of the Savitzky–Golay smoothing), and tested classification success for all combinations of k=1 to k=20 and w=5 to w=43. For simplicity we kept p, the polynomial degree for the Savitzky–Golay smoothing, fixed to 3. Once the best combination of parameters had been selected from the training set, this was then applied to the validation set and k-nn classification run using the training set to classify the spectra. Again, the classification success rate was calculated as the percentage of correct classifications. We used confusion matrices to investigate among-taxon patterns in classification success rates. The raw data and R code for running the analyses are available from figshare (Jardine et al., 2019).
FTIR spectra of the grass pollen grains (Fig. 2) show many of the same absorbance peaks as have been demonstrated in previous studies of pollen chemistry (Bağcıoğlu et al., 2015, 2017; Fraser et al., 2012, 2014; Jardine et al., 2015, 2017; Julier et al., 2016; Watson et al., 2007; Zimmermann, 2010, 2018; Zimmermann and Kohler, 2014; Zimmermann et al., 2015a, b, 2016), including a broad OH band centred on 3300 cm−1; peaks representing lipids at 2925 cm−1 (asymmetric CHn stretching), 2850 cm−1 (asymmetric CHn stretching), 1740 cm−1 (C=O stretching) and 1465 cm−1 (CH2 deformation); proteins at 1650 cm−1 (amide 1: C=O stretching) and 1550 cm−1 (amide II: combination of NH deformation and C−N stretching); and carbohydrates in the 1200 to 900 cm−1 region ( and C−OH stretching). The sporopollenin outer pollen wall (exine) is associated with peaks at 1160 and 860 cm−1, which relate to aromatic ring vibrations (C−H deformation and stretching) (Bağcıoğlu et al., 2015; Watson et al., 2007). Previously identified aromatic peaks at 1600 and 1510 cm−1 (both C=C stretching) (Bağcıoğlu et al., 2015; Jardine et al., 2015, 2017; Watson et al., 2007) are not clearly present in the FTIR spectra and may be obscured by nearby protein-related peaks. Only subtle differences among the taxa are discernible in the spectra, most obviously in the 1200 to 900 cm−1 region that relates to carbohydrates (Fig. 2). Derivatised spectra (Fig. S1 in the Supplement) show these differences more clearly, but the spectra are still highly similar among taxa.
The first two axes of a PCA on the unprocessed (i.e. z score standardised but not smoothed or differentiated) spectra account for 65 % of the variation in the dataset (Figs. 3a and S1a). Clear within-taxon groupings of samples are discernible in ordination space, although there is considerable overlap among the taxa. Most Triticum samples plot out at the lower end of axis two, along with Avena. Wild emmer and einkorn (T. dicoccoides and T. urartu, respectively) also occur close to their domesticated varieties (T. dicoccon and T.monococcum, respectively). Loading plots (Fig. 3b) show that samples occurring higher on axis one have higher peaks in the 1700 to 1000 cm−1 region, and lower values at higher wavenumbers; this may in part be driven by residual baseline effects. Axis two loadings show less of a clear signal except for a peak at 1000 cm−1, which again likely relates to variations in carbohydrates (Bağcıoğlu et al., 2015). Higher PCA axes, each successively accounting for a smaller percentage of the variation in the dataset, demonstrate further separation among taxa (Fig. S2c and e).
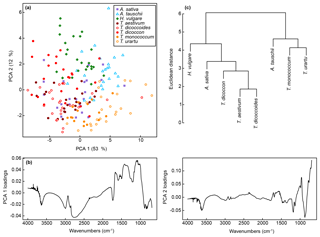
Figure 3(a) PCA of unprocessed FTIR spectra. Values in parentheses show the percentage variance explained by each PCA axis. See Fig. S2 for PCA axes three to six. (b) Loadings for PCA axes one and two. (c) Cluster analysis of unprocessed FTIR spectra, based on the mean spectrum for each species.
Table 2Maximum classification success rate on the training dataset, with the best parameter combinations from the different spectral processing approaches. k is the number of nearest neighbours in the k-nn classification algorithm, and w is the window size in the Savitzky–Golay smoothing algorithm.
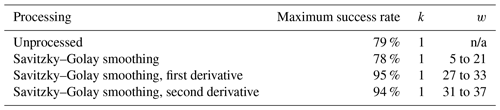
n/a – not applicable
A cluster analysis of the unprocessed taxon mean spectra (Fig. 3c) shows two main clusters. One comprises H. vulgare, Avena sativa, T. dicoccon, T. aestivum and T. dicoccoides, and the other comprises Aegilops tauschii, T. monococcum and T. urartu, with the Triticum species forming subclusters within the two main clusters. As with the PCA the wild varieties of einkorn and emmer occur close to the domesticated varieties within the cluster dendrogram.
For the training dataset, the maximum classification success rate was 95 %, with Savitzky–Golay smoothed first derivative spectra, k=1 and w=27, 29, 31 or 33 (Figs. 4 and S1a, Table 2). Misclassified samples from this combination of parameters were from T. dicoccon (four samples) and T. monococcum (two samples), with all samples from the remaining six taxa being correctly classified (Fig. 5a). The next best combination of parameters was with Savitzky–Golay smoothed second derivative spectra (94 %; Fig. S1b), then unprocessed spectra (79 %) and finally Savitzky–Golay smoothed spectra (78 %) (Fig. 4 and Table 2). In all cases the choice of k was more critical than the choice of w for maximising the classification success rate, although there was a relatively broad tolerance for both when first or second derivative spectra were used (Fig. 4).
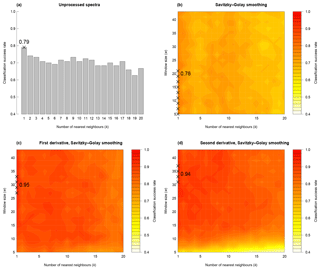
Figure 4Classification success rate with variations in number of nearest neighbours (k), smoothing window size (w) and differentiation (m). In each panel crosses show the parameter combinations with the highest classification success rate. For (b) to (d), hotter colours denote a higher classification success rate.
Based on the results from the training dataset, the validation dataset was classified using Savitzky–Golay smoothed first derivative spectra, with k=1 and w=29 (Fig. S1a). A success rate of 82 % was achieved with this combination of parameters. As with the training dataset the majority of misclassified samples were from T. dicoccon (six samples), with additional misclassifications of Avena sativa (two samples), H. vulgare (one sample), T. dicoccoides (one sample) and T. monococcum (one sample) (Fig. 5b).
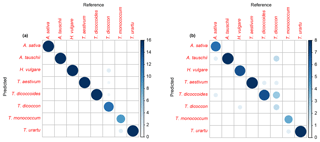
Figure 5Confusion matrices for k-nn classification. (a) Training dataset and (b) validation dataset.
A PCA of the whole dataset using the classification parameters shows a pronounced arch effect, with one gradient on axis one curving round onto axis two (Fig. 6a). Samples of H. vulgare and Aegilops tauschii occur at either end of the gradient, with samples from the other taxa appearing in overlapping groups in the middle. Together these two axes account for 45 % of the variation in the data (Fig. S2b), and as with the unprocessed data further separation among taxa occurs on higher PCA axes (Fig. S2d and f). Loading plots for axes one and two (Fig. 6b) confirm that most of the variation among spectra occurs in the fingerprint region, with differences in the 1200 to 900 cm−1 region again being important.
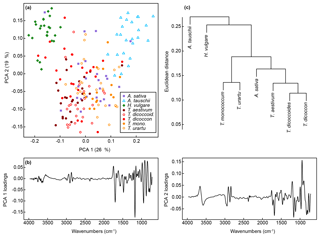
Figure 6(a) PCA of FTIR spectra processed with Savitzky–Golay smoothing and first derivatives. Values in parentheses show the percentage variance explained by each PCA axis. “T. dicoccoid.” represents T. dicoccoides; “T. mono.” represents T. monococcum. See Fig. S2 for PCA axes three to six. (b) Loadings for PCA axes one and two. (c) Cluster analysis of FTIR spectra processed with Savitzky–Golay smoothing and first derivatives based on the mean spectrum for each species.
A cluster analysis of the taxon mean spectra processed with the classification parameters (Fig. 6c) shows one cluster comprising the Triticum and Avena samples, with H. vulgare and Aegilops tauschii branching off from this. The wild varieties of einkorn and emmer occur in subclusters with the domesticated varieties within the main Triticum and Avena cluster.
Our results demonstrate a classification success rate of 95 % in the training dataset and 82 % in the validation dataset. These findings show that FTIR-based chemotaxonomy has considerable potential as a means of classifying Poaceae pollen to study grass domestication, and the spread of agriculture and landscape change over the last 10 kyr. The ability to generate species level count data from grass pollen would allow a much fuller use of the palynological record in archaeobotanical studies and would provide an additional tool to complement other lines of evidence such as grass seeds and chaff, starch grains and, more recently, DNA data from sedimentary deposits and ancient grains (Crowther et al., 2016; Fuller, 2007; Mascher et al., 2016; Piperno et al., 2004; Savard et al., 2006; Vignola et al., 2017; Weiss et al., 2004; Willcox et al., 2007).
More generally, these results show that closely related taxa can be successfully classified based on their chemical signature alone. In the present dataset this includes discriminating between ancestors and their direct descendants (e.g. T. urartu and T. monococcum; T. dicoccoides and T. dicoccon; Fig. 1b), and between parent taxa and their hybridised offspring (e.g. T. aestivum as a hybrid of T. dicoccon and Aegilops tauschii; Fig. 1b), although it was also in these cases that the majority of misclassifications occurred (Fig. 5). The decrease in classification success rate from the training dataset to the validation dataset is mostly accounted for by T. dicoccon being misclassified as T. dicoccoides, T. aestivum and Aegilops tauschii. The PCA plots (Figs. 3 and 6) also show that the T. dicoccon spectra are more widely dispersed in ordination space versus those from the other taxa, suggesting that T. dicoccon is more chemically variable than the other species considered here and therefore more challenging to classify successfully. Nevertheless, the classification accuracy demonstrated here is comparable to that of other chemical and morphological grass pollen classification studies (Julier et al., 2016; Mander et al., 2013, 2014), justifying further research in this area.
Consistent with previous studies (Julier et al., 2016; Woutersen et al., 2018), we have found that processing the FTIR spectra with smoothing and differentiation improves the classification success rate (Table 2). The taxa in this study are closely related and chemically highly similar (Fig. 2), and working with derivatised spectra (Fig. S1) allows small-scale spectral features to be enhanced for use in multivariate data exploration and classification approaches. Our results also show that not only does processing improve the separation among taxa, it also influences their relative multivariate similarity and dissimilarity, and therefore the position of taxa relative to each other in the PCA ordination and cluster analysis (Figs. 3 and 6). The processing approaches selected therefore have implications for exploring phylogenetic patterns in chemical data (Julier et al., 2016), or even using chemistry as a phylogenetic estimation tool in the fossil record. In the present case processing the spectra with Savitzky–Golay smoothing and differentiation brings all Triticum species together into one group, which makes sense from a classification and a phylogenetic point of view. However, Aegilops tauschii is then fully separated out from Triticum even though the two are very closely related, and Avena sativa is grouped in with Triticum even though it belongs to a separate tribe from all other taxa in the dataset. The presence of a phylogenetic signal in pollen chemical data, and its recoverability in FTIR spectra, whilst intriguing, requires further investigation with larger datasets.
To make this technique fully applicable to palaeoecological and archaeological settings, three relatively simple limitations in the current study will need to be addressed. First, to maximise the quality of the FTIR spectra the data here were generated on groups of grains in a 100 µm × 100 µm window, resulting in identification being made on a number of grains (8–10) rather than on the individual specimens that would need to be classified in fossil and subfossil samples. While FTIR spectra from individual pollen grains have typically suffered from scattering effects or increased noise (Zimmermann et al., 2016, 2015a; Zimmermann, 2018), this may be overcome by specific mounting approaches, such as a layer of soft paraffin between sheets of polyethylene foil (Zimmermann et al., 2016). An alternative to this methodological approach is the application of new instrumentation such as FTIR imaging systems, which combine high sample throughput with a high spatial resolution (achievable pixel resolution of ∼0.5 µm2), enabling multiple high-quality spectra to be gathered from individual pollen grains. Raman microspectroscopy might form a valuable alternative data acquisition approach, where the resolution can also be ≤2 µm and multiple measurements are obtainable from single specimens (Bağcıoğlu et al., 2015; Gottardini et al., 2007; Ivleva et al., 2005; Pummer et al., 2013; Schulte et al., 2008, 2009, 2010; Zimmermann, 2010; Zimmermann et al., 2015a).
Second, the dataset presented here was generated from fresh pollen grains that include proteins, lipids and carbohydrates, as opposed to the isolated pollen walls that are present in sedimentary deposits (Jardine et al., 2015, 2017). The success of this technique therefore needs to be tested on isolated sporopollenin, either by acetolysing the pollen grains or via other processing methods (e.g. Domínguez et al., 1998; Gonzalez-Cruz et al., 2018; Loader and Hemming, 2000; Mundargi et al., 2016). Demonstrating the success of the technique on acetolysed grains would be particularly valuable, because this would allow many existing sets of processed pollen samples to be utilised (Jardine et al., 2016). A recent FTIR study on Nitraria pollen (Woutersen et al., 2018) has shown clear species level taxonomic differentiation on chemically isolated single pollen grains, and our previous work (Jardine et al., 2015, 2016, 2017) has demonstrated that components of the sporopollenin biomacromolecule are stable after exposure to acetolysis procedures. We are therefore confident that taxonomic signals will be recoverable from processed fossil and subfossil material.
Third, in common with a number of other studies that have tested the potential for classifying pollen using chemical or morphological data (Dell'Anna et al., 2009; Holt and Bebbington, 2014; Holt and Bennett, 2014; Holt et al., 2011; Julier et al., 2016; Mander et al., 2013, 2014; Zimmermann et al., 2016; Woutersen et al., 2018), this research has focused on a relatively small dataset comprising only a few species. For this technique to be widely applied it will need to be tested on a much larger number of taxa, with each ideally being sampled from multiple individuals representing a range of environments (Holt and Bebbington, 2014), including variations in ultraviolet B (UVB) regime since this is known to influence pollen chemistry via the formation of UVB absorbing compounds (UACs) (Jardine et al., 2016; Lomax et al., 2008). This will provide a more realistic estimate of classification success when mixed, and potentially diverse, subfossil and fossil assemblages are analysed, as well as forming the basis of a chemical library that could be used as a training set for classification in archaeobotanical and palaeoecological applications.
Further enhancements to this approach may be possible by incorporating pollen grain size or surface sculpture information into the classification procedure. As already noted, the size variation across Poaceae pollen grains broadly scales with genome size, with generally larger grains in domesticated types than in wild types (Andersen, 1979; Andersen and Bertelsen, 1972; Bennett, 1972; Beug, 1961, 2004; Bottema, 1992; Firbas, 1937; Tweddle et al., 2005). If combined with chemical data, pollen grain size, along with pore and annulus size, may be useful for improving the classification of wild types and their domesticated descendants. Surface sculpturing, as already utilised by the computational image analysis classification approach of Mander et al. (2013), could together with chemistry data offer a powerful set of tools. A challenge to integrating these different data types, however, is constructing a robust and efficient workflow. FTIR imaging systems allow for measurements of grain size quite readily, but it would be considerably harder to capture SEM images of the same grains for computational feature analysis.
We have used FTIR spectroscopic data from pollen grains to demonstrate that high levels of classification success are obtainable for differentiating among domesticated grasses and their wild relatives. This approach therefore offers much potential for leveraging further information from Holocene pollen data, for reconstructing the spread of agriculture and its impact on ecosystems and environments. It also has the potential to improve on current size-based classifications of domesticated and wild grasses, or sculpture-based classifications of polyphyletic groups of taxa. Future studies need to focus on expanding the number of taxa, and working with isolated sporopollenin from single pollen grains, to provide a more realistic test of classification potential in archaeological settings.
The data and code required to run these analyses are available on figshare: https://doi.org/10.6084/m9.figshare.8046395 (Jardine et al., 2019).
The supplement related to this article is available online at: https://doi.org/10.5194/jm-38-83-2019-supplement.
PEJ, WDG, BHL, ACMJ and WTF designed the study. PEJ carried out the sampling, generated and analysed the data, and led the writing of the paper with input from WDG, BHL, ACMJ and WTF.
The authors declare that they have no conflict of interest.
We thank Mike Ambrose at the John Innes Centre for helping with pollen sampling. We also acknowledge support from the Open Access Publication Fund of the University of Münster.
This research has been supported by the Natural Environment Research Council (grant no. NE/K005294/1).
This paper was edited by Luke Mander and reviewed by two anonymous referees.
Andersen, T. S.: Identification of wild grasses and cereal pollen, Danmarks Geologiske Undersoegelse, Arbog, 1978, 69–92, 1979.
Andersen, T. S. and Bertelsen, F.: Scanning Electron Microscope Studies of Pollen of Cereals and other Grasses, Grana, 12, 79–86, https://doi.org/10.1080/00173137209428830, 1972.
Bağcıoğlu, M., Zimmermann, B., and Kohler, A.: A multiscale vibrational spectroscopic approach for identification and biochemical characterization of pollen, Plos One, 10, 1–19, https://doi.org/10.1371/journal.pone.0137899, 2015.
Bağcıoğlu, M., Kohler, A., Seifert, S., Kneipp, J., Zimmermann, B., and McMahon, S.: Monitoring of plant-environment interactions by high-throughput FTIR spectroscopy of pollen, Methods Ecol. Evol., 8, 870–880, https://doi.org/10.1111/2041-210x.12697, 2017.
Bell, B. A., Fletcher, W. J., Ryan, P., Seddon, A. W. R., Wogelius, R. A., and Ilmen, R.: UV-B-absorbing compounds in modern Cedrus atlantica pollen: The potential for a summer UV-B proxy for Northwest Africa, Holocene, 28, 1382–1394, https://doi.org/10.1177/0959683618777072, 2018.
Bennett, M. D.: Nuclear DNA Content and Minimum Generation Time in Herbaceous Plants, P. Roy. Soc. B-Biol. Sci., 181, 109–135, 1972.
Bernard, S., Benzerara, K., Beyssac, O., Balan, E., and Brown Jr., G. E.: Evolution of the macromolecular structure of sporopollenin during thermal degradation, Heliyon, 1, e00034, https://doi.org/10.1016/j.heliyon.2015.e00034, 2015.
Beug, H. J.: Leitfaden der Pollenbestimmung, Gustav Fischer Verlag, Stuttgart, 1961.
Beug, H. J.: Leitfaden der Pollenbestimmung für Mitteleuropa und angrenzende Gebiete, Pfeil, München, 2004.
Bottema, S.: Prehistoric cereal gathering and farming in the Near East: the pollen evidence, Rev. Palaeobot. Palyno., 73, 21–33, 1992.
Charmet, G.: Wheat domestication: lessons for the future, C. R. Biol., 334, 212–220, https://doi.org/10.1016/j.crvi.2010.12.013, 2011.
Christensen, B. B.: Measurement as a means of identifying fossil pollen, Danmarks Geologiske Undersøgelse (Series) IV, 3, 1–22, 1946.
Crowther, A., Lucas, L., Helm, R., Horton, M., Shipton, C., Wright, H. T., Walshaw, S., Pawlowicz, M., Radimilahy, C., Douka, K., Picornell-Gelabert, L., Fuller, D. Q., and Boivin, N. L.: Ancient crops provide first archaeological signature of the westward Austronesian expansion, P. Natl Acad. Sci. USA, 113, 6635–6640, https://doi.org/10.1073/pnas.1522714113, 2016.
Cunniff, J., Wilkinson, S., Charles, M., Jones, G., Rees, M., and Osborne, C. P.: Functional traits differ between cereal crop progenitors and other wild grasses gathered in the Neolithic fertile crescent, Plos One, 9, e87586, https://doi.org/10.1371/journal.pone.0087586, 2014.
Cushing, E. J.: Size increase in pollen grains mounted in thin slides, Pollen et Spores, 3, 265–274, 1961.
Dell'Anna, R., Lazzeri, P., Frisanco, M., Monti, F., Malvezzi Campeggi, F., Gottardini, E., and Bersani, M.: Pollen discrimination and classification by Fourier transform infrared (FT-IR) microspectroscopy and machine learning, Anal Bioanal. Chem., 394, 1443–1452, https://doi.org/10.1007/s00216-009-2794-9, 2009.
Depciuch, J., Kasprzyk, I., Drzymała, E., and Parlinska-Wojtan, M.: Identification of birch pollen species using FTIR spectroscopy, Aerobiologia, 34, 525–538, https://doi.org/10.1007/s10453-018-9528-4, 2018.
Dickson, C.: Distinguishing cereal from wild grass pollen: some limitations, Circaea, 5, 67–71, 1988.
Domínguez, E., Mercado, J. A., Quesada, M. A., and Heredia, A.: Isolation of intact pollen exine using anhydrous hydrogen fluoride, Grana, 37, 93–96, 1998.
Dvorak, J., Akhunov, E. D., Akhunov, A. R., Deal, K. R., and Luo, M. C.: Molecular characterization of a diagnostic DNA marker for domesticated tetraploid wheat provides evidence for gene flow from wild tetraploid wheat to hexaploid wheat, Mol. Biol. Evol., 23, 1386–1396, https://doi.org/10.1093/molbev/msl004, 2006.
Faegri, K. and Deuse, P.: Size variations in pollen grains with different treatment, Pollen et Spores, 2, 293–298, 1960.
Firbas, F.: Der Pollenanalytysche Nachweis des Getreidebaus, Zeitschrift für Botanik, 31, 447–478, 1937.
Fraser, W. T., Scott, A. C., Forbes, A. E. S., Glasspool, I. J., Plotnick, R. E., Kenig, F., and Lomax, B. H.: Evolutionary stasis of sporopollenin biochemistry revealed by unaltered Pennsylvanian spores, New Phytol., 196, 397-401, https://doi.org/10.1111/j.1469-8137.2012.04301.x, 2012.
Fraser, W. T., Watson, J. S., Sephton, M. A., Lomax, B. H., Harrington, G. J., Gosling, W. D., and Self, S.: Changes in spore chemistry and appearance with increasing maturity, Rev. Palaeobot. Palyno., 201, 41–46, https://doi.org/10.1016/j.revpalbo.2013.11.001, 2014.
Fuller, D. Q.: Contrasting patterns in crop domestication and domestication rates: recent archaeobotanical insights from the Old World, Ann. Bot., 100, 903–924, https://doi.org/10.1093/aob/mcm048, 2007.
Gonzalez-Cruz, P., Uddin, M. J., Atwe, S. U., Abidi, N., and Gill, H. S.: Chemical Treatment Method for Obtaining Clean and Intact Pollen Shells of Different Species, ACS Biomater. Sci. Eng., 4, 2319–2329, https://doi.org/10.1021/acsbiomaterials.8b00304, 2018.
Gottardini, E., Rossi, S., Cristofolini, F., and Benedetti, L.: Use of Fourier transform infrared (FT-IR) spectroscopy as a tool for pollen identification, Aerobiologia, 23, 211–219, 2007.
Holt, K. A. and Bebbington, M. S.: Separating morphologically similar pollen types using basic shape features from digital images: A preliminary study, Appl. Plant Sci., 2, 1400032, https://doi.org/10.3732/apps.1400032, 2014.
Holt, K. A. and Bennett, K. D.: Principles and methods for automated palynology, New Phytol., 203, 735–742, https://doi.org/10.1111/nph.12848, 2014.
Holt, K. A., Allen, G., Hodgson, R., Marsland, S., and Flenley, J.: Progress towards an automated trainable pollen location and classifier system for use in the palynology laboratory, Rev. Palaeobot. Palyno., 167, 175–183, https://doi.org/10.1016/j.revpalbo.2011.08.006, 2011.
International Wheat Genome Sequencing Consortium: A chromosome-based draft sequence of the hexaploid bread wheat (Triticum aestivum) genome, Science, 345, 1251788, https://doi.org/10.1126/science.1251788, 2014.
Ivleva, N. P., Niessner, R., and Panne, U.: Characterization and discrimination of pollen by Raman microscopy, Anal. Bioanal. Chem., 381, 261–267, https://doi.org/10.1007/s00216-004-2942-1, 2005.
Jardine, P. E. and Lomax, B. H.: Is pollen size a robust proxy for moisture availability?, Rev. Palaeobot. Palyno., 246, 161–166, https://doi.org/10.1016/j.revpalbo.2017.06.013, 2017.
Jardine, P. E., Fraser, W. T., Lomax, B. H., and Gosling, W. D.: The impact of oxidation on spore and pollen chemistry, J. Micropalaeontol., 34, 139–149, https://doi.org/10.1144/jmpaleo2014-022, 2015.
Jardine, P. E., Fraser, W. T., Lomax, B. H., Sephton, M. A., Shanahan, T. M., Miller, C. S., and Gosling, W. D.: Pollen and spores as biological recorders of past ultraviolet irradiance, Sci. Rep.-UK, 6, 1–8, https://doi.org/10.1038/srep39269, 2016.
Jardine, P. E., Abernethy, F. A. J., Lomax, B. H., Gosling, W. D., and Fraser, W. T.: Shedding light on sporopollenin chemistry, with reference to UV reconstructions, Rev. Palaeobot. Palyno., 238, 1–6, https://doi.org/10.1016/j.revpalbo.2016.11.014, 2017.
Jardine, P. E., Gosling, W. D., Lomax, B. H., Julier, A. C. M., and Fraser, W. T.: Data and code from “Chemotaxonomy of domesticated grasses: a pathway to understanding the origins of agriculture”, figshare, https://doi.org/10.6084/m9.figshare.8046395, 2019.
Joly, C., Barillé, L., Barreau, M., Mancheron, A., and Visset, L.: Grain and annulus diameter as criteria for distinguishing pollen grains of cereals from wild grasses, Rev. Palaeobot. Palyno., 146, 221–233, https://doi.org/10.1016/j.revpalbo.2007.04.003, 2007.
Julier, A. C. M., Jardine, P. E., Coe, A. L., Gosling, W. D., Lomax, B. H., and Fraser, W. T.: Chemotaxonomy as a tool for interpreting the cryptic diversity of Poaceae pollen, Rev. Palaeobot. Palyno., 235, 140–147, 2016.
Kellogg, E. A.: Relationships of cereal crops and other grasses, P. Natl. Acad. Sci. USA, 95, 2005–2010, 1998.
Köhler, E. and Lange, E.: A contribution to distinguishing cereal from wild grass pollen grains by LM and SEM, Grana, 18, 133–140, https://doi.org/10.1080/00173137909424973, 1979.
Kuhn, M.: caret: Classification and Regression Training, R package version 6.0-77, available at: https://CRAN.R-project.org/package=caret (last access: 5 June 2019), 2017.
Larson, G., Piperno, D. R., Allaby, R. G., Purugganan, M. D., Andersson, L., Arroyo-Kalin, M., Barton, L., Climer Vigueira, C., Denham, T., Dobney, K., Doust, A. N., Gepts, P., Gilbert, M. T., Gremillion, K. J., Lucas, L., Lukens, L., Marshall, F. B., Olsen, K. M., Pires, J. C., Richerson, P. J., Rubio de Casas, R., Sanjur, O. I., Thomas, M. G., and Fuller, D. Q.: Current perspectives and the future of domestication studies, P. Natl. Acad. Sci. USA, 111, 6139–6146, https://doi.org/10.1073/pnas.1323964111, 2014.
Leff, B., Ramankutty, N., and Foley, J. A.: Geographic distribution of major crops across the world, Global Biogeochem. Cy., 18, GB1009, https://doi.org/10.1029/2003gb002108, 2004.
Liland, K. H. and Mevik, B.-H.: baseline: Baseline Correction of Spectra, R package version 1.2-1, available at: https://CRAN.R-project.org/package=baseline (last access: 5 June 2019), 2015.
Loader, N. J. and Hemming, D. L.: Preparation of pollen for stable carbon isotope analyses, Chem. Geol., 165, 339–344, 2000.
Lomax, B. H., Fraser, W. T., Sephton, M. A., Callaghan, T. V., Self, S., Harfoot, M., Pyle, J. A., Wellman, C. H., and Beerling, D. J.: Plant spore walls as a record of long-term changes in ultraviolet-B radiation, Nat. Geosci., 1, 592–596, https://doi.org/10.1038/ngeo278, 2008.
Mander, L. and Punyasena, S. W.: Grass pollen surface ornamentation: a review of morphotypes and taxonomic utility, J. Micropalaeontol., 35, 121–124, https://doi.org/10.1144/jmpaleo2015-025, 2016.
Mander, L., Li, M., Mio, W., Fowlkes, C. C., and Punyasena, S. W.: Classification of grass pollen through the quantitative analysis of surface ornamentation and texture, P. Roy. Soc. B-Biol. Sci., 280, 20131905, https://doi.org/10.1098/rspb.2013.1905, 2013.
Mander, L., Baker, S. J., Belcher, C. M., Haselhorst, D. S., Rodriguez, J., Thorn, J. L., Tiwari, S., Urrego, D. H., Wesseln, C. J., and Punyasena, S. W.: Accuracy and consistency of grass pollen identification by human analysts using electron micrographs of surface ornamentation, Appl. Plant. Sci., 2, 1400031, https://doi.org/10.3732/apps.1400031, 2014.
Marcussen, T., Sandve, S. R., Heier, L., Spannagl, M., Pfeifer, M., Consortium, T. I. W. G. S., Jakobsen, K. S., Wulff, B. B. H., Steuernagel, B., Mayer, K. F. X., and Olsen, O.-A.: Ancient hybridizations among the ancestral genomes of bread wheat, Science, 345, 1250092, https://doi.org/10.1126/science.1251788, 2014.
Mascher, M., Schuenemann, V. J., Davidovich, U., Marom, N., Himmelbach, A., Hubner, S., Korol, A., David, M., Reiter, E., Riehl, S., Schreiber, M., Vohr, S. H., Green, R. E., Dawson, I. K., Russell, J., Kilian, B., Muehlbauer, G. J., Waugh, R., Fahima, T., Krause, J., Weiss, E., and Stein, N.: Genomic analysis of 6,000-year-old cultivated grain illuminates the domestication history of barley, Nat. Genet., 48, 1089–1093, https://doi.org/10.1038/ng.3611, 2016.
Meyer, R. S. and Purugganan, M. D.: Evolution of crop species: genetics of domestication and diversification, Nat. Rev. Genet., 14, 840–852, https://doi.org/10.1038/nrg3605, 2013.
Meyer, R. S., DuVal, A. E., and Jensen, H. R.: Patterns and processes in crop domestication: an historical review and quantitative analysis of 203 global food crops, New Phytol., 196, 29–48, https://doi.org/10.1111/j.1469-8137.2012.04253.x, 2012.
Meyer, D., Dimitriadou, E., Hornik, K., Weingessel, A., and Leisch, F.: e1071: Misc Functions of the Department of Statistics, Probability Theory Group (Formerly: E1071), TU Wien, R package version 1.6-8, available at: https://CRAN.R-project.org/package=e1071 (last access: 5 June 2019), 2017.
Mundargi, R. C., Potroz, M. G., Park, J. H., Seo, J., Tan, E. L., Lee, J. H., and Cho, N. J.: Eco-friendly streamlined process for sporopollenin exine capsule extraction, Sci. Rep.-UK, 6, 19960, https://doi.org/10.1038/srep19960, 2016.
Pappas, C. S., Tarantilis, P. A., Harizanis, P. C., and Polissiou, M. G.: New Method for Pollen Identification by FT-IR Spectroscopy, Appl. Spectrosc., 57, 23–27, 2003.
Petersen, G., Seberg, O., Yde, M., and Berthelsen, K.: Phylogenetic relationships of Triticum and Aegilops and evidence for the origin of the A, B, and D genomes of common wheat (Triticum aestivum), Mol. Phylogenet. Evol., 39, 70–82, https://doi.org/10.1016/j.ympev.2006.01.023, 2006.
Piperno, D. R., Weiss, E., Holst, I., and Nadel, D.: Processing of wild cereal grains in the Upper Palaeolithic revealed by starch grain analysis, Nature, 430, 670–673, 2004.
Preece, C., Livarda, A., Wallace, M., Martin, G., Charles, M., Christin, P. A., Jones, G., Rees, M., and Osborne, C. P.: Were Fertile Crescent crop progenitors higher yielding than other wild species that were never domesticated?, New Phytol., 207, 905–913, https://doi.org/10.1111/nph.13353, 2015.
Preece, C., Livarda, A., Christin, P. A., Wallace, M., Martin, G., Charles, M., Jones, G., Rees, M., and Osborne, C. P.: How did the domestication of Fertile Crescent grain crops increase their yields?, Funct. Ecol., 31, 387–397, https://doi.org/10.1111/1365-2435.12760, 2017.
Preece, C., Clamp, N. F., Warham, G., Charles, M., Rees, M., Jones, G., and Osborne, C. P.: Cereal progenitors differ in stand harvest characteristics from related wild grasses, J. Ecol., 106, 1286–1297, https://doi.org/10.1111/1365-2745.12905, 2018.
Pummer, B. G., Bauer, H., Bernardi, J., Chazallon, B., Facq, S., Lendl, B., Whitmore, K., and Grothe, H.: Chemistry and morphology of dried-up pollen suspension residues, J. Raman. Spectrosc., 44, 1654–1658, https://doi.org/10.1002/jrs.4395, 2013.
R Core Team: R: A language and environment for statistical computing, Vienna, Austria, R Foundation for Statistical Computing, 2017.
Reitsma, T. J.: Size modification of recent pollen grains under different treatments, Rev. Palaeobot. Palyno., 9, 175–202, 1969.
Rowley, J. R.: The Exine Structure of “Cereal” and “Wild” Type Grass Pollen, Grana Palynologica, 2, 9–15, https://doi.org/10.1080/00173136009429441, 1960.
Savard, M., Nesbitt, M., and Jones, M. K.: The role of wild grasses in subsistence and sedentism: new evidence from the northern Fertile Crescent, World Archaeol., 38, 179–196, https://doi.org/10.1080/00438240600689016, 2006.
Schulte, F., Lingott, J., Panne, U., and Kneipp, J.: Chemical characterization and classification of pollen, Anal. Chem., 80, 9551–9556, https://doi.org/10.1021/ac801791a, 2008.
Schulte, F., Mäder, J., Kroh, L. W., Panne, U., and Kneipp, J.: Characterization of Pollen Carotenoids with in situ and High-Performance Thin-Layer Chromatography Supported Resonant Raman Spectroscopy, Anal. Chem., 81, 8426–8433, 2009.
Schulte, F., Panne, U., and Kneipp, J.: Molecular changes during pollen germination can be monitored by Raman microspectroscopy, J. Biophotonics, 3, 542–547, https://doi.org/10.1002/jbio.201000031, 2010.
Sluyter, A.: Analysis of maize (Zea mays subsp. mays) pollen: normalizing the effects of microscope-slide mounting media on diameter determinations, Palynology, 21, 35–39, 1997.
Soreng, R. J., Peterson, P. M., Romaschenko, K., Davidse, G., Zuloaga, F. O., Judziewicz, E. J., Filgueiras, T. S., Davis, J. I., and Morrone, O.: A worldwide phylogenetic classification of the Poaceae (Gramineae), J. Syst. Evol., 53, 117–137, https://doi.org/10.1111/jse.12150, 2015.
Steemans, P., Lepot, K., Marshall, C. P., Le Herisse, A., and Javaux, E. J.: FTIR characterisation of the chemical composition of Silurian miospores (cryptospore and trilete spores) from Gotland, Sweden, Rev. Palaeobot. Palyno., 162, 577–590, 2010.
Stevens, A. and Ramirez-Lopez, L.: An introduction to the prospectr package, R package Vignette, R package version 0.1.3, available at: https://cran.r-project.org/web/packages/prospectr/vignettes/prospectr-intro.pdf (last access: 5 June 2019), 2013.
Strömberg, C. A. E.: Evolution of Grasses and Grassland Ecosystems, Ann. Rev. Earth Pl. Sc., 39, 517–544, https://doi.org/10.1146/annurev-earth-040809-152402, 2011.
Tweddle, J. C., Edwards, K. J., and Fieller, N. R. J.: Multivariate statistical and other approaches for the separation of cereal from wild Poaceae pollen using a large Holocene dataset, Veg. Hist. Archaeobot., 14, 15–30, https://doi.org/10.1007/s00334-005-0064-0, 2005.
Varmuza, K. and Filzmoser, P.: Introduction to Multivariate Statistical Analysis in Chemometrics, CRC Press, Boca Raton, 336 pp., 2009.
Venables, W. N. and Ripley, B. D.: Modern Applied Statistics with S, Springer, New York, 2002.
Vignola, C., Masi, A., Balossi Restelli, F., Frangipane, M., Marzaioli, F., Passariello, I., Stellato, L., Terrasi, F., and Sadori, L.: δ13C and δ15N from 14 C-AMS dated cereal grains reveal agricultural practices during 4300–2000 BC at Arslantepe (Turkey), Rev. Palaeobot. Palyno., 247, 164–174, https://doi.org/10.1016/j.revpalbo.2017.09.001, 2017.
Watson, J. S., Septhon, M. A., Sephton, S. V., Self, S., Fraser, W. T., Lomax, B. H., Gilmour, I., Wellman, C. H., and Beerling, D. J.: Rapid determination of spore chemistry using thermochemolysis gas chromatography-mass spectrometry and micro-Fourier transform infrared spectroscopy, Photochem. Photobiol., 6, 689–694, https://doi.org/10.1039/b617794h, 2007.
Wei, T. and Simko, V.: R package “corrplot”: Visualization of a Correlation Matrix, R package version 0.84, available at: https://github.com/taiyun/corrplot (last access: 5 June 2019), 2017.
Weiss, E., Wetterstrom, W., Nadel, D., and Bar-Yosef, O.: The broad spectrum revisited: evidence from plant remains, P. Natl. Acad. Sci. USA, 101, 9551–9555, https://doi.org/10.1073/pnas.0402362101, 2004.
Willcox, G., Fornite, S., and Herveux, L.: Early Holocene cultivation before domestication in northern Syria, Veg. Hist. Archaeobot., 17, 313–325, https://doi.org/10.1007/s00334-007-0121-y, 2007.
Woutersen, A., Jardine, P. E., Bogotá-Angel, G., Zhang, H.-X., Silvestro, D., Antonelli, A., Gogna, E., Erkens, R. H. J., Gosling, W. D., Dupont-Nivet, G., and Hoorn, C.: A novel approach to study the morphology and chemistry of pollen in a phylogenetic context, applied to the steppe-desert taxon Nitraria L. (Nitrariaceae), PeerJ, 6, e5055, https://doi.org/10.7717/peerj.5055, 2018.
Zimmermann, B.: Characterization of Pollen by Vibrational Spectroscopy, Appl. Spectrosc., 64, 1364–1373, 2010.
Zimmermann, B.: Chemical characterization and identification of Pinaceae pollen by infrared microspectroscopy, Planta, 247, 171–180, https://doi.org/10.1007/s00425-017-2774-9, 2018.
Zimmermann, B. and Kohler, A.: Infrared spectroscopy of pollen identifies plant species and genus as well as environmental conditions, Plos One, 9, 1–12, https://doi.org/10.1371/journal.pone.0095417.t001, 2014.
Zimmermann, B., Bagcioglu, M., Sandt, C., and Kohler, A.: Vibrational microspectroscopy enables chemical characterization of single pollen grains as well as comparative analysis of plant species based on pollen ultrastructure, Planta, 242, 1237–1250, https://doi.org/10.1007/s00425-015-2380-7, 2015a.
Zimmermann, B., Tkalčec, Z., Mešić, A., and Kohler, A.: Characterizing aeroallergens by infrared spectroscopy of fungal spores and pollen, Plos One, 10, 1–22, https://doi.org/10.1371/journal.pone.0124240, 2015b.
Zimmermann, B., Tafintseva, V., Bağcıoğlu, M., Høegh Berdahl, M., and Kohler, A.: Analysis of Allergenic Pollen by FTIR Microspectroscopy, Anal. Chem., 88, 803–811, https://doi.org/10.1021/acs.analchem.5b03208, 2016.